OpenAI’s ChatGPT is everywhere nowadays and offers benefits like faster resume screening or interview question generation. But, for its speed and convenience, HR professionals should know that ChatGPT’s outputs are riddled with hidden biases. While ChatGPT bias is not a popular talking point, it does exist.
Bloomberg’s team of researchers experimented with using fictitious names and resumes to measure algorithmic bias and hiring discrimination. They used GPT to generate eight different resumes, edited them to show the same level of educational attainment, years of experience, and job title, and associated them with men or women who are either Black, White, Hispanic, or Asian. This is just one example of ChatGPT bias.
The result: for a Financial Analyst job opening, GPT ranked the resume with an Asian woman’s name at the top position. While the resume with a Black woman’s name at the bottom, indicating racial ChatGPT bias.
The experiment was repeated 1,000 times using hundreds of different names and combinations. It uncovered that resumes with Black American names were least likely at the top rank for the financial analyst role.
The experiment was repeated for 4 job postings—HR business partner, senior software engineer, retail manager, and financial analyst. It found that resumes labeled with names distinct from Black Americans were the least likely to be ranked as the top candidates for financial analyst and software engineer roles.
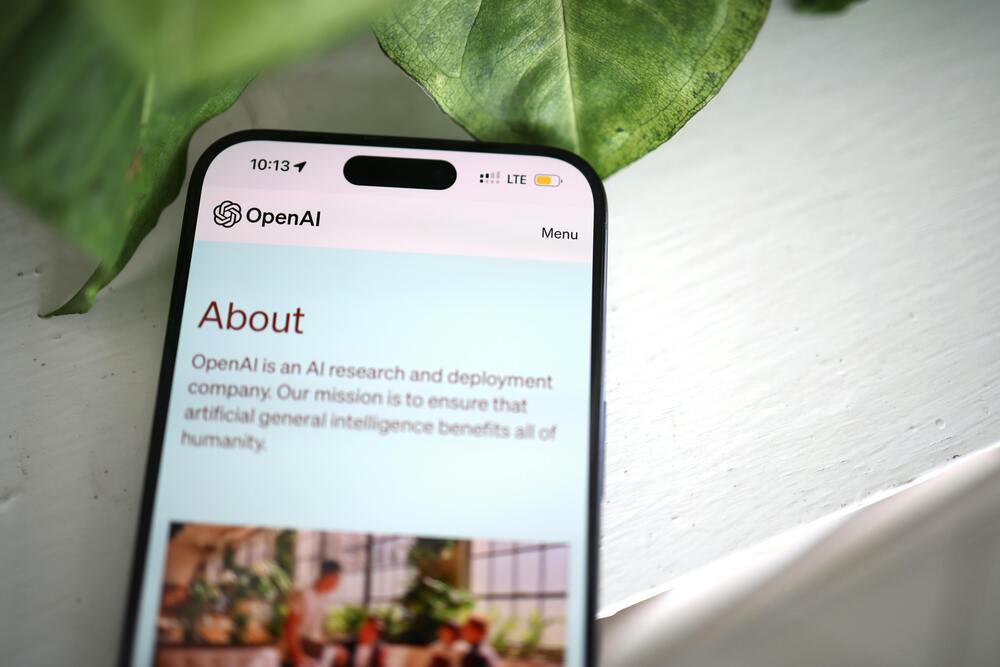
Examples of Bias in ChatGPT
ChatGPT has different biases that can significantly impact HR decision-making, just like many AI models.
Here are the most common ChatGPT bias examples HR professionals need to know:
1. Data Bias/Algorithmic Bias
It’s the root cause of many AI biases. When the data you used to train ChatGPT is biased, you will also generate biased outputs.
For instance, if your data has demographics or historical prejudices, ChatGPT will also have the same biases.
2. Confirmation Bias
ChatGPT may produce responses that are aligned with existing stereotypes or beliefs held by its developers or the data set on which it was trained. Or it may prioritize details that confirm existing biases.
For example, if asked what makes good leaders and ChatGPT responded that women make excellent leaders because research has shown that women often excel in leadership due to their strong communication skills, empathy and ability to collaborate effectively with others.
While the response is accurate and acknowledges research findings, it may overlook or downplay alternative evidence that challenges the assumption that all women are inherently good leaders.
ChatGPT’s response may unintentionally perpetuate confirmation bias. Similarly, it may contribute to the reinforcement of stereotypes about gender and leadership.
3. Associative Bias
This happens when ChatGPT makes unfair connections based on patterns in the data.
For example, ChatGPT responded that a good leader should be assertive, confident, and decisive, like a CEO or military general when asked about the qualities of a good leader.
ChatGPT has associated qualities of a good leader with stereotypical roles such as CEO or military general, reflecting common associations present in its training data, resulting in this ChatGPT bias.
While this may be correct, it may undervalue other important leadership characteristics like empathy, emotional intelligence, and collaboration, which are not usually linked with traditional hierarchies.
4. Availability Bias
ChatGPT might overemphasize readily available information. The concepts or ideas may influence the information in its training data without considering their relevance or importance.
For instance, if you asked ChatGPT about the common reasons for employee turnover, it may reply that poor management, lack of career development opportunities, and low salary are factors that may be frequently emphasized in turnover discussions. While these factors are indeed important contributors, they may overlook other significant elements like work-life balance, culture, or job satisfaction.
5. In-Group Bias
This ChatGPT bias can emerge if the developers of ChatGPT belong to a specific demographic. The tool might favor candidates or approaches that resonate more with the developers’ experiences.
For example, it may favor approaches or communication styles that resonate more with the developers’ experiences. If the developers primarily came from a sales background, ChatGPT might unconsciously be in favor of candidates with strong sales skills for other positions as well.
How do these biases apply to HR situations?
1. Recruitment
Job ads and screening criteria may inadvertently reinforce stereotypes about certain demographic groups, leading to biased candidate selection. For example, resumes for engineering roles historically used more masculine terminology. It may downplay resumes with a more neutral or feminine language, overlooking qualified candidates.
If certain ethnic groups are underrepresented leadership positions, it might unfairly rank candidates from those backgrounds lower for leadership roles.
If a candidate has a recent accomplishment highlighted on their profile, this qualification might be prioritized over deeper but less recent experiences. This could disadvantage candidates with longer careers or those with gaps in their resumes due to caregiving responsibilities or transitioning careers.
Applicants from certain universities or previous employers with higher performers might rank higher. This is likely based on their educational background or work history. Thus, unfairly ranking qualified individuals from diverse backgrounds.
If ChatGPT was trained to prioritize specific skills or experiences that align with their own background (e.g., a strong educational background), it might favor candidates with similar profiles. Thus, ignoring job seekers with transferable skills or those with equally valuable skills and experiences from different backgrounds.
2. Performance Management
If performance evaluation tools use data with historical biases (e.g., men historically rated higher for assertiveness), it can unfairly disadvantage women.
Let’s say a manager has an implicit bias against remote workers. When they review an employee working from home using ChatGPT, it might focus on minor missed deadlines and downplay major achievements.
If the system prioritizes recent projects, it might overlook an employee’s significant contributions in previous quarters.
And, if ChatGPT-generated feedback perpetuates existing stereotypes about certain groups’ abilities or behavior, it could negatively affect performance evaluations, leading to unfair or inaccurate assessments of employee performance
Evaluation bias can happen If ChatGPT-generated feedback is based on criteria that systematically favor or put into disadvantage some employees based on their demographic characteristics or backgrounds.
3. Training and Development
If Chat-GPT training data associates certain ethnicities with specific skill sets, it might recommend development programs that reinforce stereotypes, which limit opportunities for diverse employees.
In the event that training suggestions are based on past employee details, it might recommend programs that reinforce existing stereotypes. These include leadership training for men and communication skills for women.
Training materials curated by ChatGPT could subconsciously associate leadership qualities with certain communication styles, it might overlook employees with different communication styles who are equally qualified for leadership roles.
4. Compensation
If salary data used for making compensation decisions has historical gender pay gaps, ChatGPT might recommend lower salaries for women even if their performance is equal.
Let’s say ChatGPT prioritizes an employee’s most recent salary when deciding compensation. It could disadvantage an employee with a long history within the company but whose salary lagged behind due to previous under-negotiation or lack of promotions in a disadvantageous position.
If ChatGPT prioritizes an employee’s latest performance data when making compensation recommendations, it could undervalue an employee with a solid track record of strong performance which might have had a recent setback
Should the ChatGPT developers suggest a benefits package based on past employee selections, it might favor benefit options that resonate more with the developers’ needs. Thus, overlooking the needs of the diverse workforce.
5. Promotions
When reviewing employees eligible for promotions, ChatGPT may highlight recent accomplishments, potentially overlooking valuable contributions from the previous period. So if the employee doesn’t have many achievements currently, they may rank lower compared to colleagues who may have recent wins but have average past performance
When evaluating candidates for promotion, ChatGPT bias might show up by favoring those who exhibit traits similar to past successful leaders’ or developers’ leadership styles. So, it may overlook individuals with different leadership qualities.
6. Employee Relations
ChatGPT may not fully understand the emotional context of employee concerns.
For instance, in a discrimination complaint it might prioritize keywords over the employee’s full narrative. It might also give preference to certain genders or ethnicities in leadership positions. Or it may favor specific employee groups, leading to unfair treatment of employees from outside groups.
How to mitigate ChatGPT bias in HR situations
1. Eliminate ChatGPT bias
To avoid ChatGPT bias, when using it for HR tasks, train it on a dataset that reflects workplace diversity. This covers a wide range of demographic backgrounds, experiences, and perspectives to ensure that the model makes fair and unbiased predictions and recommendations:
- Demographic diversity – different races, ethnicities, genders, ages, socioeconomic backgrounds, and geographic locations
- Experiential diversity – different levels of education, employment histories, career paths, and life events
- Perspective diversity – different opinions, beliefs, cultural norms, values, and societal norms from various communities and cultures
2. Enforce bias detection and monitoring strategies
First, research common biases in AI, like social bias towards certain genders in leadership or in-group bias reflecting the training data. Then, mechanisms will be implemented to detect and track any ChatGPT bias.
When using ChatGPT, frame prompts carefully to counter potential biases. For example, instead of asking for “strong leadership qualities,” ask for “demonstrated ability to motivate and guide teams effectively.”
3. Create policies and guidelines
Establish rules governing AI use in HR settings. These rules should address ChatGPT issues like fairness, privacy, and accountability. Include guidelines on how to handle these biases properly:
- Fairness – ensure AI systems treat all employees fairly and equitably
- Transparency – provide explanations for the model’s predictions or recommendations. Disclose information about data sources, model architecture, and decision-making criteria
- Accountability – define the roles and responsibilities of the HR staff involved in deploying AI. Implement auditing and evaluation processes to ensure compliance with ethical guidelines
4. Treat ChatGPT as an adviser, not as the final decision-maker
HR professionals should use ChatGPTs as a starting point. The human resource department should always review and refine any ChatGPT recommendations for any biases before implementing them. For instance, if it suggests focusing heavily on the educational background of a specific school, consider other approaches.
Remember, make decisions based on human judgments and established regulations. Hiring, promotions, and disciplinary actions should always be decided by trained HR staff. For example, you can use it to identify potentially biased language in job descriptions. Or analyze anonymized employee feedback to detect trends, but always involve HR professionals in interpreting the results to avoid bias.
5. Use ChatGPT for HR tasks that are less prone to biases
For instance, you can implement blind resume screening. ChatGPT can analyze resumes for keywords and skills instead of names, schools, or universities, reducing bias toward these factors.
Or ask standardized job interview questions focusing on job requirements to ensure a fair evaluation for all candidates. Instead, employ ChatGPT for tasks less prone to bias, like scheduling or generating reports based on factual employee data.
6. Regularly monitor for changes and updates
As ChatGPT is updated with new data, its biases might change. Continuously evaluate and refine the AI model to address emerging biases and improve performance.
This includes gathering user feedback, monitoring data distribution changes, and updating the model accordingly.
Why I wrote this:
Ongig aims to inform HR professionals about the latest developments in HR technology, including AI and ChatGPT. ChatGPT helps recruiters to automate HR functions. As the technology is constantly evolving, HR should be guided accordingly. To help streamline writing inclusive job descriptions, check out Ongig. Our software uses generative AI, like ChatGPT, plus offers suggestions to remove bias at the same time. Contact us for a demo.
Shout-Outs:
Open AI’s GPT is a Recruiter’s Dream Tool. Test Show there’s Racial Bias – Bloomberg