- Innovative Resume Ideas to Help You Stand Out - May 8, 2025
- How to Create an ATS Career Page That Converts: Tips & Tools - April 29, 2025
- 5 Helpful Examples of a SHRM Job Description Template - March 4, 2025
AI software has become a quintessential aspect of modern hiring and recruitment. These intuitive solutions have led to cost-effective hiring outcomes for enterprise recruitment teams. With AI, employers can hone in on the most qualified talent with personalized recruitment strategies while automating repetitive administrative workflows. So, with that comes a new set of AI related words and terminology that we all must get to know.
Yet, with great power comes great responsibility – the responsibility of keeping up with AI terminologies that is! So, it isn’t a surprise to find team members struggling with all the AI talk flooding pantries, water coolers, and meeting rooms these days.
We at Ongig hear you! To that end, we have compiled a handy dandy list of frequently used AI related words and recruitment terms (and sample use cases for clarity to boot). So your crew can hop in any AI recruitment discussion like a pro.
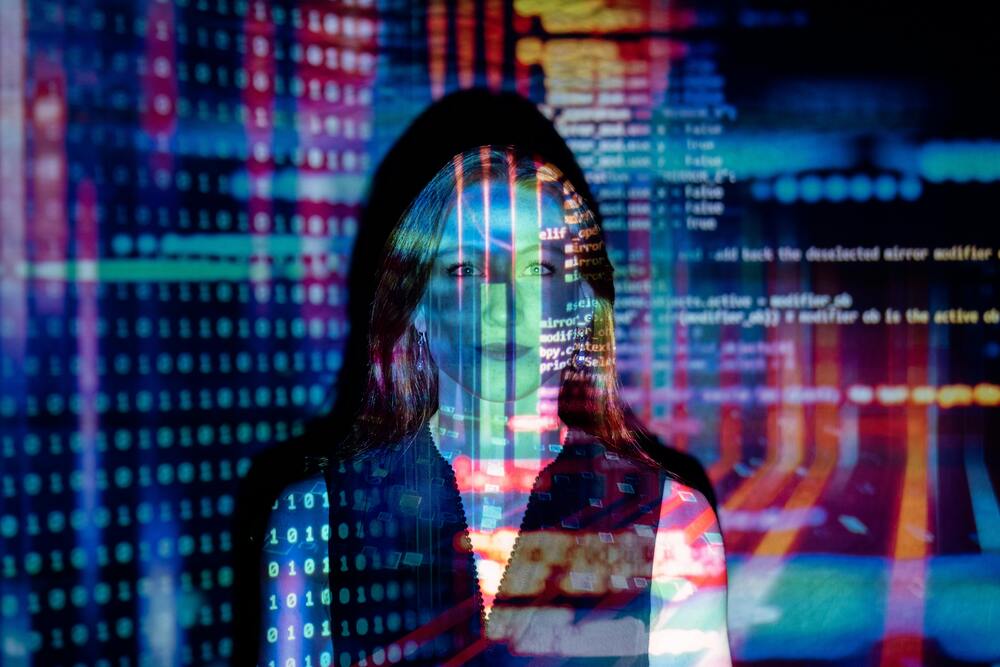
Check back often as we update the list regularly to keep up with the AI pace. Also, here are 13 Best Must-Read Books on AI in Recruitment for the enthusiasts among us seeking further reading. Now, on to the glossary of AI related words!
AI Application Development
AI application development is a specialized branch of computer science that optimizes user experiences with carefully researched algorithms. Talent acquisition app developments include proprietary recruitment chatbots and mobile job portals for enhancing candidate experiences.
Use case: The tech-savvy HR team dedicated a decade of candidate experience data to AI application development, resulting in a user-friendly chatbot with 24/7 availability.
AI Hallucination
AI hallucinations are mistakes or inaccuracies in a model’s generated response. Recruitment AI hallucinations may include perpetuated biases due to exclusionary training data.
Use case: The company’s internal IT team prevents risky recruiting AI hallucinations by ensuring regular training data audits.
Algorithms
Algorithms provide AI talent acquisition programs with specific instructions for automating recruitment tasks. So, AI algorithms could include simple or complex functions for analyzing and processing candidate information. For instance, an AI recruitment solution could apply clustering algorithms to detect minute differences between large volumes of data sets.
Use case: Ongig’s Text Analyzer applies an advanced AI algorithm that automatically detects and replaces biased JDs with compelling alternatives that appeal to qualified hires.
Applicant Tracking System (ATS)
Innovative applicant tracking systems (ATS) use AI technology to sort and manage candidate information. So, an AI-supported ATS empowers recruiting teams to manage large volumes of applicants cost-effectively. It does this by ranking, sorting, and also tracking each candidate profile.
Use case: Research shows that 97.4 percent of Fortune 500 companies used a detectable ATS in streamlining their recruitment processes.
Artificial General Intelligence (AGI)
Artificial general intelligence is a theoretical version of AI. It involves proposed software functions that can exactly mimic the completion of any human task while surpassing natural cognitive abilities. Therefore, AGI solutions can learn and adapt information independently beyond the predetermined parameters that limit traditional AI.
Use case: The future of recruitment AI could include AGI components that supplement hiring teams with additional talent acquisition support through improved creativity and problem-solving.
Artificial Intelligence (AI)
Artificial Intelligence (AI) is the umbrella term that describes the sophisticated technology behind automated hiring and recruitment solutions. AI technology empowers recruiters with actionable talent acquisition insights from data sources like industry reports, governmental statistics, and job seeker trends.
Use case: Implementing AI tools in the recruitment pipeline minimizes talent biases and contributes to workplace diversity through improved skills-based hiring.
Artificial Neural Network (ANN)
Artificial neural network in machine learning functions similarly to the neuronal connections in the human brain. So, an artificial neural network powers machine learning techniques through links between data nodes in a multi-layered AI structure resembling the biological brain.
Use case: Data science research shows that AI’s artificial neural networks reduce inherent hiring prejudices, promoting diversity, inclusion, and ethics in the workplace.
Bias Mitigation
AI recruitment technology’s bias mitigation features eliminate inherent biases in an organization’s recruitment practices. Specifically, computer programs fitted with bias mitigation tools detect traces of bias in human language that might repel qualified candidates.
Use case: Our hiring managers rolled out the machine learning framework for bias mitigation, which vetted and refined JDs according to objective training data.
Big Data
Big data refers to a large quantity of information generated, collected, and processed by AI. This is often compiled from diverse sources. AI professionals characterize big data with the five Vs of velocity, volume, value, variety, and veracity. Also, AI’s accessibility to big data enables hiring teams to achieve time-effective recruitment decisions.
Use case: The insightful big data comprising candidate profiles, job market trends, and workforce reports have boosted the response of talent-sourcing channels.
Chatbots
Chatbots are AI-supported programs that automate hiring and recruitment processes through precise algorithms. An advanced recruitment chatbot usually features specialized AI functions like predictive analytics and natural language processing (NLP). So, it carries human-like conversations that benefit initial interview stages involving high volumes of job applicants.
Use case: Recruiters have dramatically reduced time-to-hire by implementing robust chatbots that enrich the candidate experience.
Cognitive Computing
Cognitive computing is a type of AI capability that mimics how the human mind comprehends, reasons, and responds to language. Therefore, an advanced human resource information system (HRIS) could combine contextual awareness, machine learning, and natural language processing (NLP) to apply cognitive computing in candidate interactions.
Use case: Chatbots equipped with cognitive computing time-effectively managed first-stage interviews so human recruiters could focus on refining talent outreach strategies.
Computer Vision
Candidate screening AI tools like HireVue employ computer vision technology to process and analyze images during recruitment. For instance, computer vision can support resume parsing or video interview analytics to infer actionable insights from subtle visual cues.
Use case: The AI reviewed asynchronous video interviews with computer vision, improving the culture fit of hires based on nonverbal cue observations.
Data Mining
Data mining enables AI users to discover complex patterns and hidden information from data sets. So, recruiting teams could use data mining to extract job seeker trends and industry remuneration standards to boost application rates.
Use case: The company’s hiring managers raised the quality of hire through data mining by analyzing candidate CVs and guiding job matches.
Data Science
The field of data science combines analytics, AI, and other subject matter expertise (e.g., recruitment strategies) to identify actionable insights from a company’s organizational data. For instance, AI solutions would leverage historical data and employee performance to guide accurate predictive analytics for improved hiring outcomes.
Use case: Data science-driven recruitment solutions can enhance skills-based hiring through role-specific assessments and reaching out to underserved communities.
Data Set
A data set in AI refers to the collection of data treated collectively as a single unit by the program. Therefore, data sets in an AI system enable it to learn and process logical patterns with greater efficiency. Examples of data sets in recruitment AI include candidate job experiences and job descriptions.
Use case: Company X trained their recruitment AI with data sets of successful hires’s background information to fine-tune the candidate selection process for the best fit.
Deep Learning Models
AI recruitment systems that employ deep learning models apply complex, labeled data in completing specific tasks. Examples of these models in recruitment include applicant tracking systems and skills assessment platforms.
Use case: Ongig’s Text Analyzer platform utilizes deep learning models to diversify talent pools by eliminating biases and poor formatting in JD content.
Generative Adversarial Network (GAN)
A recruitment AI’s generative adversarial network (GAN) is an advanced class of machine learning framework for managing generative AI.
These AI systems power unsupervised learning through two main steps. GAN creates neural networks of generators (i.e., synthetic data) and discriminators that distinguish synthetic from real-world data. So, the comparison continuously improves the learning, efficiency, and accuracy of the AI recruitment model’s generative functions.
Use case: Modern recruitment software suite may include GAN that formulates realistic scenarios for relevant skill assessments to determine top hires for a time-sensitive job vacancy.
Generative AI
Generative AI is a subcategory of AI technology that optimizes recruiter efficiencies by generating quality content based on user prompts. The technology generates content through AI learning patterns based on current data, resulting in various output possibilities.
Potential applications of generative AI include resume screening and creating impactful JD content. For instance, teams can apply Ongig’s Text Analyzer software to generate unbiased and compelling JDs at scale for quicker time-to-fill.
Use case: Our marketing agency’s creative team of recruiters utilized generative AI to create candidate-specific job ads that filled the most time-sensitive vacancies.
Generative Pre-Trained Transformer (GPT)
A generative pre-trained transformer puts the GPT in the widely popular ChatGPT AI model. GPT is a type of large language model (LLM) that uses deep learning to fulfill specific tasks. So, AI recruiters apply GPT for hiring use cases including resume screening, chatbot interactions, and applicant sourcing.
Use case: The GPT chatbot optimizes applicant engagement through conversational AI achieved through the latest natural language processing (NLP) advancements.
Historical Data
Historical data in AI refers to relevant information collected from past events, user interactions, and circumstances. The data may include labor market trends and economic trajectories. It may also include job seeker input like popular keywords. Therefore, predictive AI recruitment systems tap into historical data to generate quality TA content such as JDs and career site FAQs.
Use case: Our trusted HRIS consults a vast pool of industry-specific historical data to streamline inclusive recruitment initiatives while complying with the latest DEIB standards.
Hyperautomation
Hyperautomationr is the AI-driven strategy of managing complex workflows through automated tools and systems. These may involve a blend of machine learning, NLP, and robotics process automation.
Use case: Recruiters save valuable time and effort by introducing more hyperautomation elements to their hiring workflows.
Image Recognition
Certain AI recruitment models like Jobscan implement image recognition to identify and categorize visual elements. For instance, a resume parsing AI tool could use image recognition to highlight the latest jobseeker keywords, skills, and experience from CVs in image format. Advanced image recognition solutions may include algorithms that correct low-quality images for improved results.
Use case: The latest version of the AI-driven ATS includes an image recognition tool that interprets valuable applicant information from scanned resumes.
Information Retrieval
Information retrieval refers to the speed of identifying and acquiring relevant information from (at times unstructured and messy) data environments.
AI systems efficiently conduct information retrieval from large datasets via specific algorithms. Recruiters can leverage AI to expedite repetitive administrative tasks (while minimizing error) that involve information retrieval such as scheduling interviews and vetting JDs.
Use case: AI has made information retrieval cost-effective for recruitment teams so they can focus on high-level decision-making and strategic hiring.
Input Data
An AI recruitment model uses input data to shed light on a specific problem, situation, or request. The information may come in various forms including labeled or raw data. So, common examples of recruitment input data include candidate resumes, job competency assessment results, and recorded video interviews.
Use case: Recruiters integrate an assortment of input data in their AI-supported ATS to streamline the candidate selection for the urgent job vacancy.
Knowledge Base
An AI system’s knowledge base refers to its complex library of information needed to perform specific tasks. A quality AI recruitment solution features a comprehensive knowledge base that improves TA processes like shortlisting candidates. It also conducts cultural fit assessments and schedules interview follow-ups.
Use case: The ATS’s expansive knowledge base included insights into a candidate’s background, employment history, past salary details, and contact information.
Large Language Models (LLMs)
Advanced AI solutions may comprise large language models (LLMs). This is a pre-trained AI program that processes vast amounts of candidate data for accelerating talent acquisition. So, an LLM-supported recruitment solution empowers employers to tailor candidate engagement. It does this by reviewing entire job profiles and resumes rather than relying on keywords.
Use case: Our applicant tracking system utilizes LLM to automate complex interactions throughout the recruitment pipeline with timely and applicant-specific communication.
Machine Learning
Machine learning is a subset of artificial intelligence systems, where advanced recruitment solutions retain actionable insights from training data. So, hiring companies can use the data to strengthen candidate engagement through conversational chatbots, improved resume parsing, and analyzed video interviews.
Use case: The innovative F&B enterprise boosted talent acquisition in Q3 with a machine learning AI solution that matched candidate profiles with the criteria of open positions.
Machine Learning Model
An AI’s machine learning model refers to a specific algorithm that detects and responds to data patterns within a system. For instance, hiring teams can apply machine learning models in their ATS for efficiently screening resumes and discovering the right job fit for a specific industry or role.
Use case: Our ATS solution used the logistic regression machine learning model to hire top-performing talent based on a candidate’s industry experience and in-demand skill set.
Natural Language Processing (NLP)
Natural language processing is an AI category that enables programs to recognize and extract subtle contextual cues from natural human language, and mimic expressions.
Rather than relying on preset data points like predictive analytics, NLP software applications such as top-tier chatbots can improve candidate engagement. So, it does this by checking automated responses for linguistic details like inherent employer biases and the latest DEIB buzzwords.
Use case: The recently launched candidate analytics solution uses NLP to observe and respond to nonverbal cues throughout the interview process.
Pattern Recognition
Pattern recognition is a form of data analysis performed by AI machine learning models. An AI recruitment solution could apply pattern recognition to improve job matches according to candidate profile information such as employer history and educational background.
Use case: Enterprise recruiters use AI’s pattern recognition functions to highlight key JD sections and keywords that attract quality hires.
People Analytics
People analytics refers to the AI data for managing the human aspects of a company. HR teams can leverage people analytics to resolve lingering talent issues such as recruitment bottlenecks and employee disengagement.
Use case: The AI processing of people analytics empowered our talent managers in maximizing engagement and hiring while reducing turnover rates.
Predictive AI Analytics
Predictive AI is an emerging technology that empowers recruiters with past hiring/recruitment data that forecast job market and talent acquisition trends. As such, hiring teams can enhance decision-making to fill talent gaps despite tight market conditions.
Use case: Our HR manager applied predictive AI analytics to improve application rates with talent sourcing according to anticipated career and role challenges.
Prompt Engineering
Automated recruitment solutions often include prompt engineering that provides specific instructions for improving the results of generative AI or large language models. So, the process improves candidate experiences and conversion through thoughtfully composed AI prompts that prioritize job seeker needs.
Use case: Our company’s diligent software engineers implemented prompt engineering updates that garnered an overwhelming candidate response for our recent recruitment campaign.
Reinforcement Learning
Reinforcement learning is a category of machine learning that enables AI systems to optimize cumulative rewards. As such, AI recruitment systems fitted with reinforcement learning can exceed the functions of predicting outcomes or deciphering logical patterns. So, in these scenarios, the AI system, known as an agent, proactively receives feedback to gradually improve output data.
Use case: The AI-supported HRIS features reinforcement learning, which constantly improves the quality of candidate experience based on applicant engagement data.
Search Engines
AI-driven search engines like Perplexity AI and Bing apply processes like natural language processing and advanced algorithms that identify user intent. As such, these search engines provide recruiters with a deeper understanding of candidates for optimal engagement.
Use case: The most reliable candidate sourcing strategies include detailed analytics acquired from AI search engines.
Supervised Learning
Recruitment AI with supervised learning uses labeled datasets in predicting and analyzing information. The machine learning method involves the straightforward process of matching an input with an output information.
Use case: The prototype of our AI ATS interpreted labeled candidate information grouped into hired or unhired subsets.
Synthetic Data
Synthetic data refers to any data generated by simulations or algorithms rather than from tangible real-world sources. So, recruiters and hiring organizations may access synthetic data that mimics actual events or trends if a situation involves data inaccessibility or scarcity.
Use case: Due to a lack of real-world information on hand, we decided to train our chatbots using synthetic data to engage and convert top talent with conversational AI.
Sentiment Analysis
Recruitment AI with sentiment analysis capabilities combines data functions like natural language processing and biometrics. So these functions work to uncover the underlying emotional factors behind candidate experience. These analyses may also include emotional verbal responses or non-verbal cues that suggest a candidate’s long-term suitability for a role or organization.
Use case: AI-supported hiring teams rely on sentiment analysis to discover exclusive talent acquisition insights from candidate surveys and remote interviews.
Text Analyzer
Ongig’s Text Analyzer is a cloud-based AI recruitment tool that automatically generates and optimizes JDs according to organizational hiring objectives. The AI platform also integrates with an in-house applicant tracking system (ATS) and human resource information system (HRIS) for smooth onboarding.
Use case: Enterprise hiring teams can speed up talent acquisition with scalable and standardized JDs by applying Text Analyzer to their recruitment campaigns.
Training Data
An AI’s training data refers to the user input information that establishes and continuously develops learning models. The quality of AI training data is critical for maintaining fair and efficient recruitment workflows. Errors or hallucinations within training data could result in far-reaching consequences such as perpetuating employer biases or overlooking top talent.
Use case: A lack of regular auditing and transparency led to undiscovered inaccuracies in the AI’s training data resulting in untapped talent pools.
Turing Test
The Turing Test is a philosophical and technological assessment, also known as the Imitation Game, created by Alan Turing, widely regarded as the founding father of computer science. So, AI experts apply the Turing Test as a technical inquiry to check if a machine’s responses differ from human-generated answers.
Use case: According to data engineers, the organization’s new recruitment chatbot powered by ChatGPT-4 generated human-like conversations that passed the Turing Test with flying colors.
Unsupervised Learning
Modern AI models equipped with unsupervised learning models can independently label and group new information without human intervention/explicit instructions.
So, these advanced solutions comprise algorithms that enable them to learn from unlabelled data by interpreting patterns and relationships within the information. As such, unsupervised learning emulates the dynamic learning process within the human brain.
Use case: The recruitment software tapped on unsupervised learning to identify and group candidates according to similar skill sets and experiences for a given role.
Zero-Shot Learning (ZSL)
An AI system equipped with handling zero-shot learning scenarios can complete tasks without labeled training examples. So, in machine learning, AI with zero-shot learning identifies core data attributes and categorizes them into new examples/classes of information based on context.
As such, recruitment AI with zero-shot learning can facilitate TA efforts by matching candidates to a newly established job position despite the lack of past recorded employee experiences.
Use case: The company’s recently deployed recruitment AI model uses ZSL to anticipate and resolve TA issues based on predictive data assessments.
Why I Wrote This?
The Ongig team strongly believes in the limitless possibilities of AI recruitment. Our Text Analyzer platform also uses advanced algorithms to create winning JDs that convert your top-performing job seekers. So, request a Text Analyzer demo to discover how you can optimize talent acquisition with data-backed consistency!
Shout-Outs:
- Korn Ferry – AI in Recruiting 2024: Pros and Cons
- Carnegie Mellon University – Generative AI for Organizational Behavior: Use Cases of Generative AI in Talent Recruitment
- By Recroot.io, LinkedIn – AI-Driven Predictive Analytics in Recruitment
- By Rock Content Writer – Artificial Intelligence Algorithm: Everything You Need To Know About It
- Oracle – What Is AI Model Training & Why Is It Important?
- Indeed – Pros and Cons of Recruiting Chatbots
- By Kelsey Purcell, Jobscan – 2024 Applicant Tracking System (ATS) Usage Report: Key Shifts and Strategies for Job Seekers
- By Dave Bergmann, IBM – What is zero-shot learning?
- IBM – What Are Large Language Models (LLMs)?
- IBM – What is Unsupervised Learning?
- Label Your Data – What Is a Dataset in Machine Learning: The Complete Guide
- By Jim Holdsworth, IBM – What is Data Mining?
- Coursera – What Are the 5 Vs of Big Data?
- By Nitika Sharma, Analytics Vidhya – What is Cognitive Computing: Definition, Features, Application, Challenges and Opportunities
- Standford Encyclopedia of Philosophy – The Turing Test
- AWS – What is AGI (Artificial General Intelligence)?
- McKinsey & Company – What is prompt engineering?
- Machine Learning Mastery – A Gentle Introduction to Generative Adversarial Networks (GANs)
- By Ivan Belcic and Cole Stryker, IBM – What is GPT (generative pretrained transformer)?
- IBM – What is hyperautomation?